
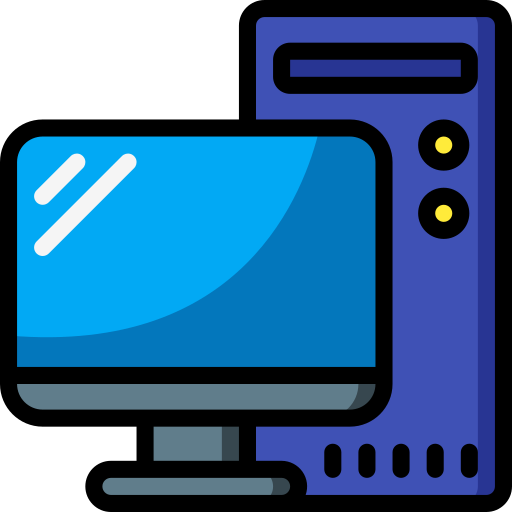
Oh good to know.
It used to be awful but I’m glad to hear it’s improving.
Oh good to know.
It used to be awful but I’m glad to hear it’s improving.
Maybe snapdrop?
When I was obsd I did FTP and rsync for everything. Syncthing had dinner performance issues for me.
Maybe Seafile but I had a bad time with that.
From memory MTP is pretty flaky and quite slow.
ADB push is pretty good but at that stage rsync
is just as easy.
Put SSH in the phone and you can do it all from the computer too.
Lisp is fun, and recursion can be slick. it is hard to read though!
Are you able to buy unlocked directly from Google? I typically avoid the carrier when I can.
The whole notion of LSP has been nice.
An LLM is an equation, fundamentally. Map a word to a number, equation, map back to words and now llm. If you’re curious write a name generator using torch with an rnn (plenty of tutorials online) and you’ll have a good idea.
The parameters of the equation are referred to as weights. They release the weights but may not have released:
Open source is typically more concerned with the open nature of the code base to foster community engagement and less on the price of the resulting software.
Curiously, open weighted LLM development has somewhat flipped this on its head. Where the resulting software is freely accessible and distributed, but the source code and material is less accessible.
The energy use isn’t that extreme. A forward pass on a 7B can be achieved on a Mac book.
If it’s code and you RAG over some docs you could probably get away with a 4B tbh.
ML models use more energy than a simple model, however, not that much more.
The reason large companies are using so much energy is that they are using absolutely massive models to do everything so they can market a product. If individuals used the right model to solve the right problem (size, training, feed it with context etc. ) there would be no real issue.
It’s important we don’t conflate the excellent progress we’ve made with transformers over the last decade with an unregulated market, bad company practices and limited consumer Tech literacy.
TL;DR: LLM != search engine
Yeah I did the smart thing and walked away from research.
Total dead end, Industry don’t care at all.
I use it a lot when I’m writing my notes (ie joplin/obsidian), I’ll use flux or stable diffusion for a few iterations until I can create an image that Is consistent with what I’m writing.
It can be really convenient to be able to recognize an image as you’re browsing through notes that are otherwise just filled with code or maybe a recount of the day.
I’m sure most consumers consider excessive use of generative AI to be in bad form. It certainly doesn’t exude professionalism.
Oh, okay, I understand what you’re saying now.
Yeah, I don’t trust any of the VPN providers. There’s just no evidence that they’re trustworthy. I reach for Tor (or i2p sometimes).
I typically run all the torrenting stuff in a container, I’ve never actually used that VPN to browse. I just spin the container up and down when I want my bandwidth back.
I’ve had a good experience with AirVPN. I mean, I only use it for torrenting, but… Is there a good reason not to go with them for torrents?
To be fair, wireguard is pretty painless.
Absolutely that’s what the internet was made for!
But family photos keep a bit more secure, Particularly if it’s syncing directly from your phone, I take a lot of explicit photos of my wife, but also code that I’m writing on my computer, or the kids playing, etc.
Keepass with rsync
/ unison
or a local git server works pretty well too.
Airvpn has port forward i believe.
I don’t actually dislike ai imagery, I think it can produce interesting imagery. However, I must concede that is an excessive use of boilerplate bog-standard AI imagery.
I don’t think I would have made too much of a difference because the state-of-the-art models still aren’t a database.
Maybe more recent models could store more information in a smaller number of parameters, but it’s probably going to come down to the size of the model.
The Only exception there is if there is indeed some pattern in modern history that the model is able to learn, but I really doubt that.
What this article really calls to light is that people tend to use these models for things that they’re not good at because it’s being marketed contrary to what it is.
I think they all would have performed significantly better with a degree of context.
Trying to use a large language model like a database is simply A misapplication of the technology.
The real question is if you gave a human an entire library of history. Would they be able to identify relevant paragraphs based on a paragraph that only contains semantic information? The answer is probably not. This is the way that we need to be using these things.
Unfortunately companies like openai really want this to be the next Google because there’s so much money to be hired by selling this is a product to businesses who don’t care to roll more efficient solutions.
Mobile offline sync is a lost cause. The dev environment, even on Android, is so hostile you’ll never get a good experience.
Joplin comes close, but it’s still extremely unreliable and I’ve had many dropped notes. It also takes hours to sync a large corpus.
I wrote my own web app using Axum and flask that I use. Check out dokuwiki as well.